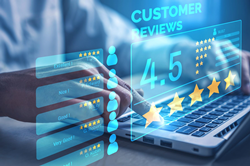
New research co-authored with others by two Saunders College of Business faculty researchers provides one method for searching out five-star fakes. (Shutterstock)
ROCHESTER, N.Y. (PRWEB)
November 21, 2022
It doesn’t have to be the holidays for Amazon to receive a lot of attention from product buyers, but business on the nation’s largest online retailer is especially brisk this time of year.
While the company says it has over 12,000 employees who look for fraud on its site, the growing glut of fake reviews leaves one wondering whether that device or accessory you’re considering is truly so highly rated.
New research co-authored with others by Ali Tosyali and Gijs Overgoor, assistant professors in the Department of MIS, Marketing, and Analytics at RIT’s Saunders College of Business, provides one method for searching out five-star fakes.
In the study, titled, “Detecting Fake Review Buyers Using Network Structure: Direct Evidence from Amazon,” Tosyali and Overgoor:
-
Employed network science and machine learning to develop a highly accurate method for detecting fake reviews. - Revealed that products that purchase fake reviews share a common set of reviewers, predominantly among Facebook groups, which are highly clustered in a product-reviewer network.
- Claim their research method is highly accurate and, unlike approaches relying on reviews’ text, their approach is more resistant to manipulation by sellers.
The research, featured in The Wall Street Journal earlier this year, offers a promising means of helping consumers avoid being duped by these fraudulent and misleading reviews. The paper has been published on pnas.org.
The two RIT professors became involved in the research after Tosyali’s personal experience purchasing a vitamin on Amazon. “The product I purchased had many reviews—approximately 5,000—with an average rating of 4.9,” Tosyali recalled. “After using it, I observed some side effects and returned the product.”
Upon returning the product, Tosyali looked at the reviews more closely and saw many negative comments, which referenced the side effects he experienced.
“However, because of the large number of five-star reviews, the product looked pretty satisfactory,” Tosyali observed. “At that point, I wondered if there was a way to detect fake reviews that mislead customers.”
During a follow-up conversation with Overgoor, Tosyali mentioned his experience and the two Saunders College faculty decided to look into the issue more closely.
Overgoor later discovered that some UCLA colleagues had initiated a paper analyzing the market of fake reviews using a dataset of Amazon reviews, and the researchers agreed to collaborate.
The dataset used took about nine months to collect, with the help of UCLA undergrads who infiltrated Facebook groups where fake reviews are solicited. After identifying sellers and products for which faux reviews were gathered, all reviews were then combined together. The research took about a year, employing techniques from network science, machine learning, and text and image analysis.
Based on their research, Tosyali and Overgoor offer the following tips for consumers to spot fake Amazon reviews.
- Compared to products not buying fake reviews, products that purchase fake reviews are highly connected and clustered in the network, implying that products buying fake reviews tend to have more reviewers in common than other products.
- While regular products receive their reviews from a dispersed set of millions of Amazon customers, products purchasing fake reviews must rely on the relatively small number of reviewers participating in the “fake review marketplace.”
- Features derived from the product-reviewer network are especially useful for detecting fake reviews because—in addition to being more predictive than text or metadata features—they are more difficult or costly to manipulate by sellers than text or review timing.
According to the RIT researchers, e-commerce platforms can use the research “as a flagging mechanism,” and “the research increases customers’ awareness of these harmful initiatives.”
“They could be more vigilant about five-star reviews and be aware that five-star reviews could be paid,” Tosyali said.
The team is working on additional research on this topic, Overgoor added.
Share article on social media or email: